–
Room P3.10, Mathematics Building
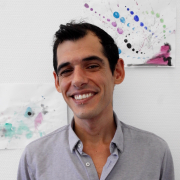
Miguel Couceiro, Université de Lorraine
Galois theory of analogical classifiers
Analogical proportions are statements of the form "a is to b as c is to d", denoted a:b::c:d) where a, b, c, d are tuples of attribute values describing items. Analogical inference relies on the idea that if four instances a, b, c, d are in analogical proportion for most of the attributes describing them, then it may still be the case for the other attributes. Similarly, if class labels are known for a, b, c and unknown for d, then one may infer the label for d as a solution of an analogical proportion equation.
Theoretically, it is quite challenging to characterize situations where such an analogical inference principle (AIP) can be soundly applied. In case of Boolean attributes, a first step for explaining the analogical mechanism was to characterize the set of classifiers for which AIP is sound (i.e., no error occurs). At IJCAI 2017, we took the minimal model of analogy (i.e., containing only patterns of the form x : x :: y : y and x : y :: x : y) and showed that these analogical classifiers coincide with the set of affine Boolean functions. Moreover, when the function is close to being affine, we showed at IJCAI 2018 that the prediction accuracy remains high. These results were then extended at SUM 2020 to nominal domains.
However, the notion of analogy preservation gives rise to a Galois connection between classifiers and the models of analogy that they preserve. In this talk, we will explore this polarity and establish a Galois theory of analogical classifiers. We will also derive several consequences and, if time allows, we will discuss recent applications in NLP related tasks.
Most of the results that will be presented constitute ongoing research work being developed with Erkko Lehtonen, Esteban Marquer and Pierre-Alexandre Murena, Nicolas Hug, Henri Prade and Gilles Richard.